Business Need
Mathesis Labs to develop a predictive analytics solution for predicting equipment (fixed & mobile equipment) failure in ball mill gearboxes.
Business Benefits
Solution Highlights
Data Analysis Inputs:
- Analyzed mill-related data, data sheets, system overviews, drawings, photos, FMEA reports, and O & M Manuals.
- Utilized standard task procedures, including alignment, etc.
Condition Monitoring:
- Analyzed MHM analysis reports & history, lube analysis reports, and external condition monitoring reports.
- Incorporated process and condition monitoring data from OSIPI and SAP-PM history.
Failure History Identification:
Identified failure history, including chipped gear tooth, gear & pinion bearing failures, pipe resonance, etc.
Data Normalization:
Normalized 157 parameters spread across separate flat files for efficient analysis.
Data Analysis Approach:
Applied slice and dice techniques while analyzing failures with contextual links to the condition data across all shared reports.
Root Cause Analysis:
- Utilized Cause & Effect Diagram for comprehensive root cause analysis.
- Conducted engineering analysis of gear & pinion failures, considering factors such as vibration, lubrication, misalignment, etc.
Failure Mode Effects & Criticality Analysis (FMECA):
Identified predictor variables and performed FMECA to enhance understanding of failure modes.
Fatigue Propagation Techniques:
Applied fatigue propagation techniques at the system level, considering root cause, sub-system degradation, and failure propagation.
Prediction Model Building:
Built a robust prediction model to predict gearbox failure based on analyzed data.
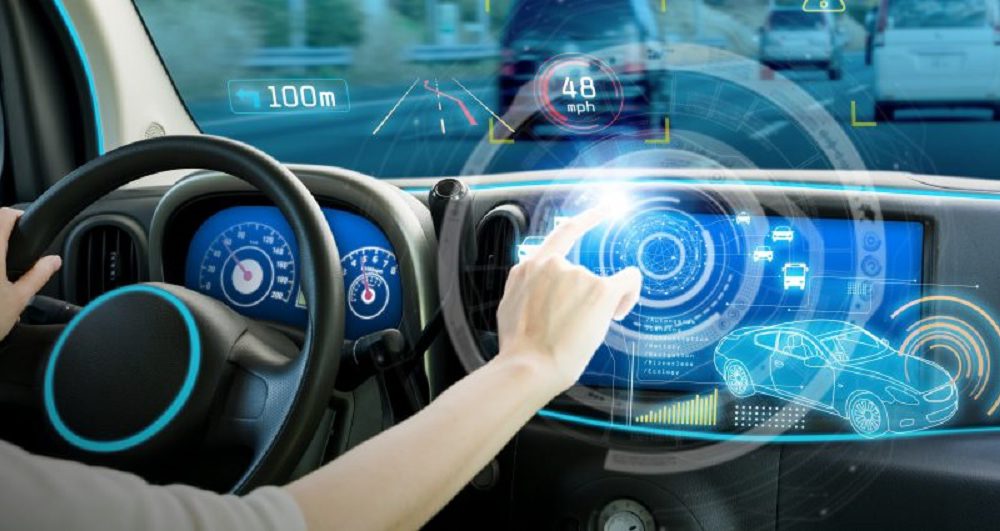
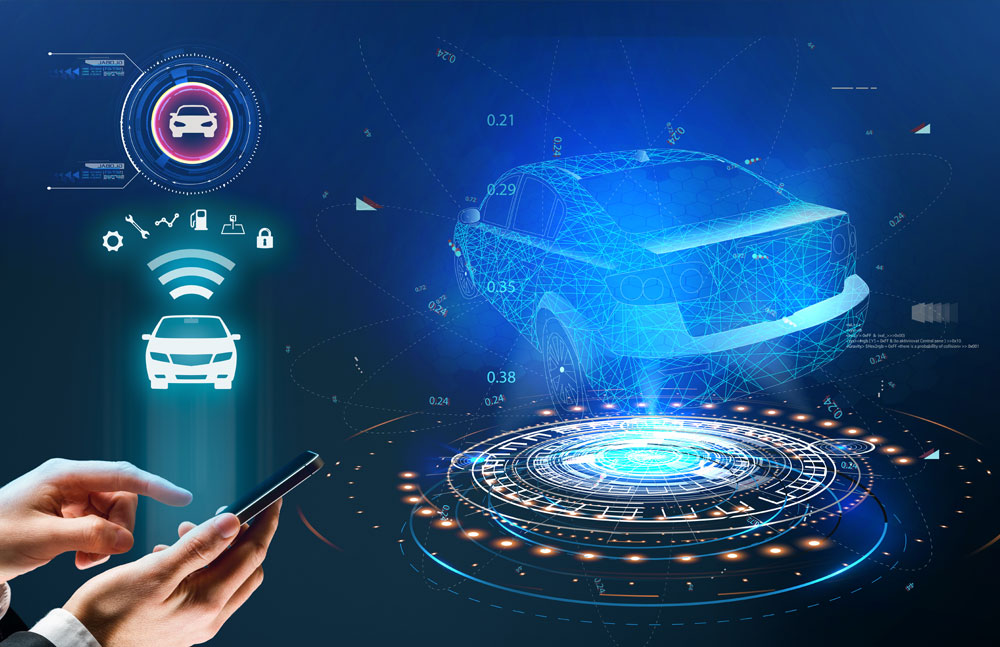
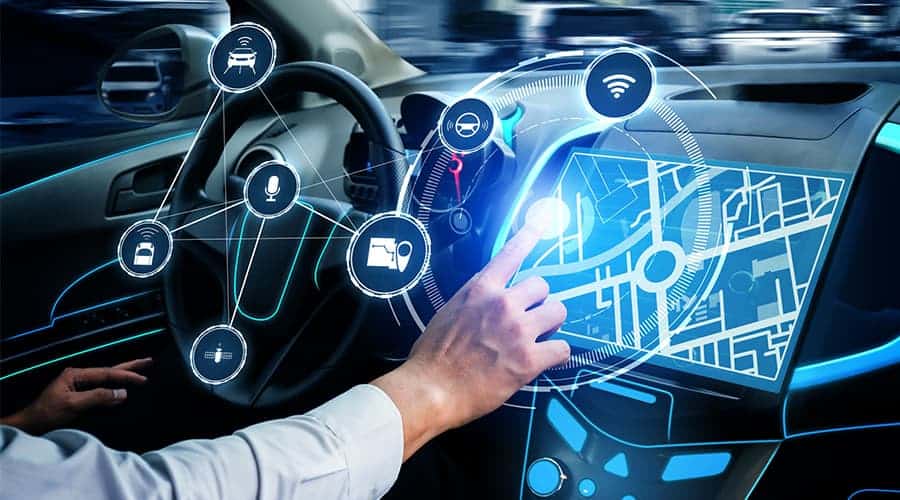
Benefits
Life Prediction and Health Index:
Provided life prediction of the gearbox and machine health degradation indexes for proactive maintenance.
Cost Savings:
Achieved over 20% savings on maintenance costs through predictive maintenance strategies.
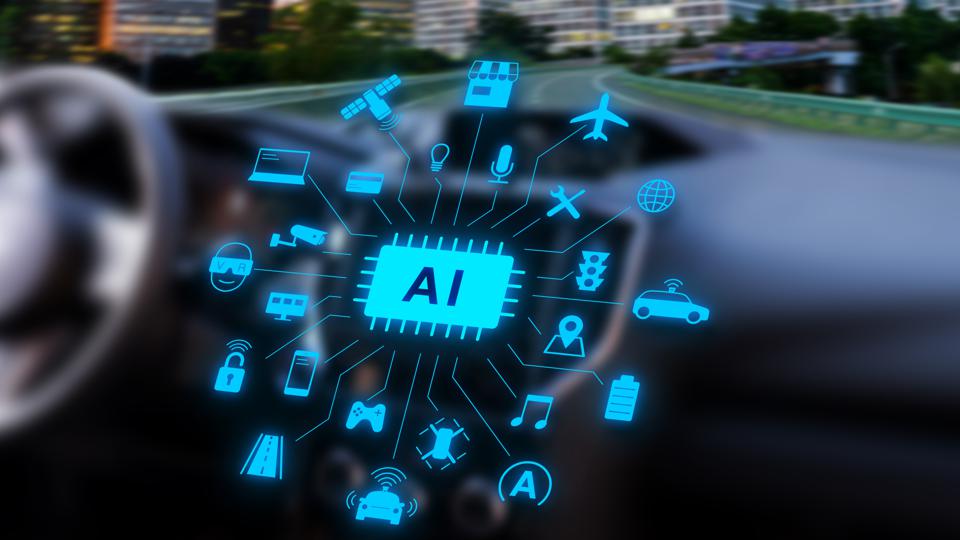
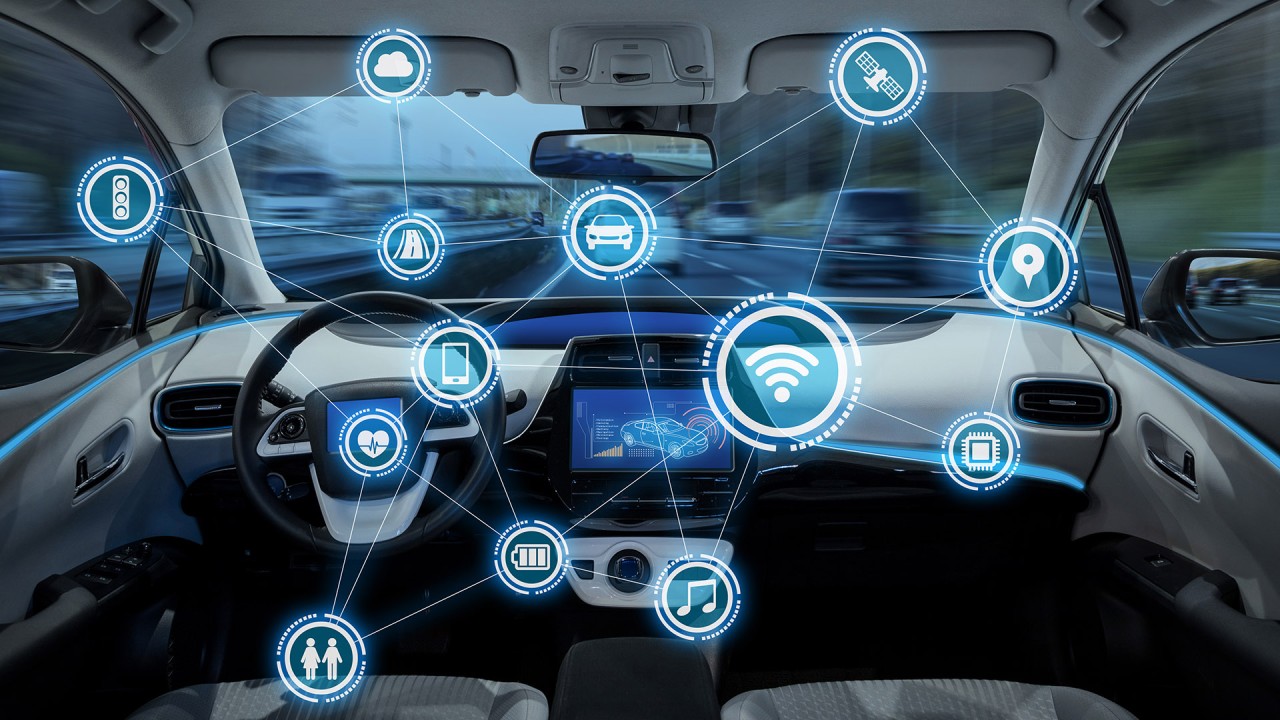
The Results
Mathesis Labs’ predictive analytics solution revolutionizes ball mill gearbox maintenance, offering a data-driven approach to equipment health management. The comprehensive analysis, root cause identification, and predictive modelling contribute to substantial cost savings and improved operational efficiency.