Objective
Mathesis Labs aimed to elevate spot welding quality by predicting defective spots through continuous monitoring of process parameters. Additionally, the goal was to forecast trends and potential breaches of threshold limits, providing insights for informed decision-making.
Methodology
Mathesis Labs implemented predictive models leveraging Support Vector Machines (SVM) for defect prediction, integrating process parameters and inspection results. For forecasting process parameters, the Autoregressive Integrated Moving Average (ARIMA) model was employed.
Business Benefits
Tools/Technology
Microsoft R Server: Utilized for statistical analysis and predictive modelling.
Microsoft Power BI: Empowered the creation of interactive and visually rich dashboards for data visualization.
Microsoft SQL Server: Served as the robust database management system for storing and managing large volumes of process data.
Benefits Achieved
Defect Prediction Accuracy: The implemented defect prediction model successfully identified over 75% of faulty welds, contributing to improved product quality.
Reduced Manufacturing Time: The near real-time detection of defects in the spot welding process significantly decreased the overall manufacturing time by 25%. This accelerated response minimizes delays and enhances operational efficiency.
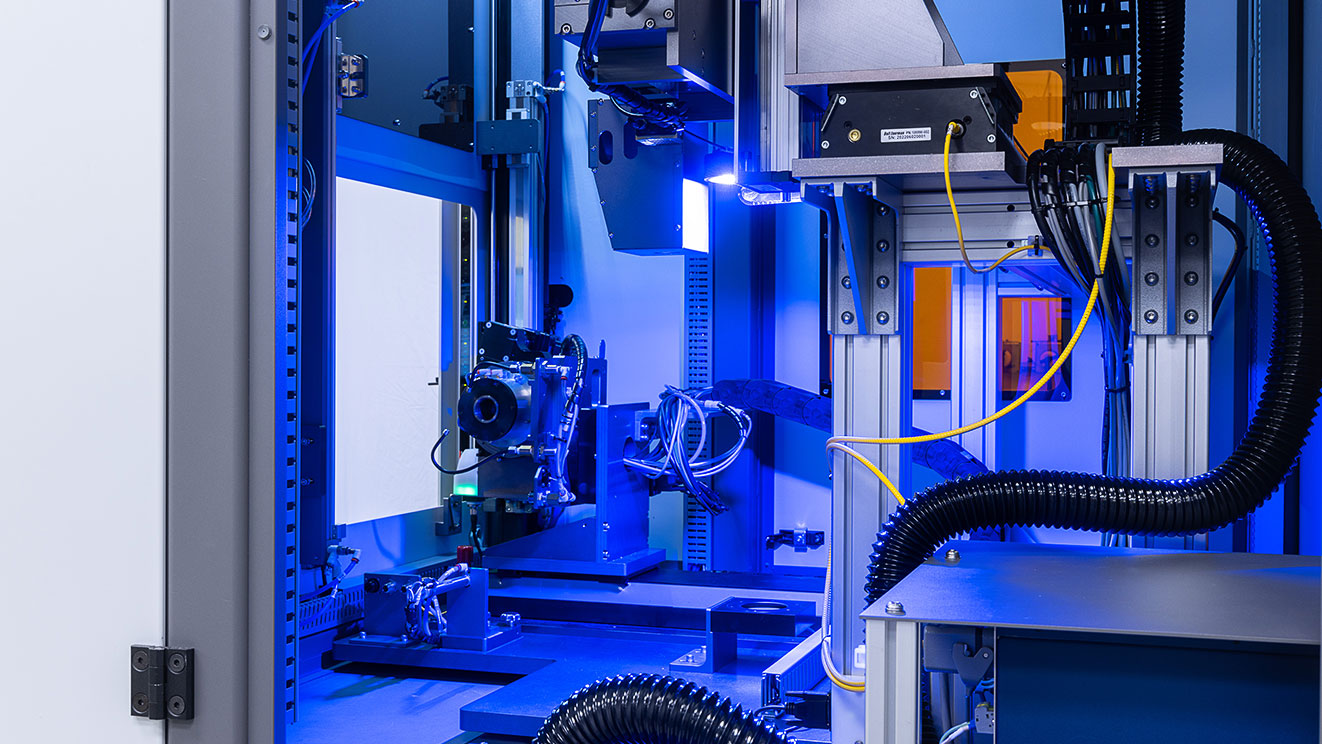
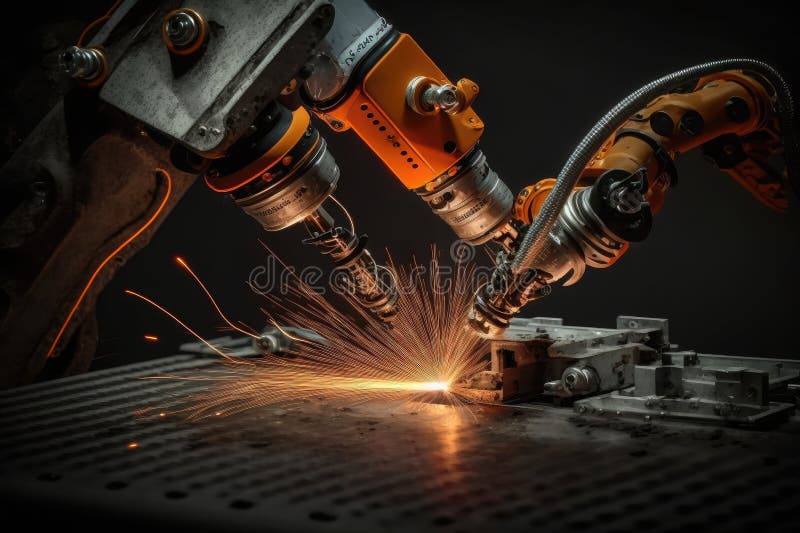
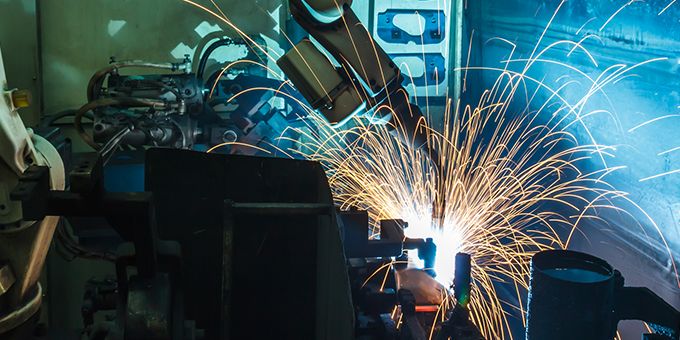
The Results
By combining advanced analytics, machine learning models, and visualization tools from the Microsoft ecosystem, Mathesis Labs achieved substantial improvements in defect identification and manufacturing efficiency, ultimately enhancing the quality of spot-welded products.